- Scientists at Flinders University in Australia have developed a machine-learning model that predicts which species are at risk of extinction if another species is removed from an ecosystem or an invasive one is introduced.
- Trained on data on how species interact with each other, the model could serve to alert conservation managers on which vulnerable species to focus on, the developers say.
- They tested the model successfully in Australia’s Simpson Desert, where it accurately predicted which species invasive foxes and cats preyed on.
- However, the shortage of data on species interactions, along with the possible biases that arise, are gaps that still need to be filled in the model.
About 100 years ago, a predator control program at Yellowstone National Park drove the wolves native to the region extinct. That kicked off the gradual deterioration of the entire ecosystem. Without wolves, the elk population exploded, leading to overgrazing. Without trees and plants of adequate size, beavers were unable to make dams. This in turn affected the flow of water in local rivers, which eventually impacted the fish.
Had this ecological cascade occurred in 2023, an artificial intelligence model might have been able to predict the repercussions well in advance.
A machine-learning model developed by scientists at Flinders University in Australia can predict which species are likely to go extinct if a predator or prey is introduced or removed from an ecosystem. It’s trained on data on how different species interact with each other.
A study published in the journal Ecography delineates the framework for how to collate data on species interactions and train machine-learning algorithms to predict extinction cascades — the secondary extinctions that occur as part of a ripple effect of primary extinctions in an ecosystem.

The model is based on the important correlation between species interactions and the health of ecosystems. For ecosystems around the world to maintain a healthy equilibrium, it’s important to not disrupt the complex food webs that exist within it.
“Many extinctions that have happened in the past, and that will happen in the future, happen through species interactions,” John Llewelyn, lead author of the study and research fellow in paleoecological network modeling at Flinders University, told Mongabay in a video interview. “Another example is when you look at invasive species moving to a new area. An introduced predator could prey on native species, and so those interactions are important to predict so that you can prioritize their conservation.”
In 2021, Llewelyn and his team started collecting data on how different species interacted with each other. For each of those species, they also gathered data on their traits that would help determine their position in the food web. This included data on body size, diet (do they eat plants? If not, do they eat vertebrates or invertebrates?), the time of the day when they’re active (diurnal, nocturnal or crepuscular?), and their habitats (canopy or shrubs or ground level?). Once the team had trained the algorithm, they could then “hand it a list of other species with their traits and ask the model ‘Who is going to eat who out of that list?’,” Llewelyn said.
To corroborate the model’s efficacy, Llewelyn tested it out at the Simpson Desert in Australia, for which he already had detailed predator-prey data.
“We actually predicted predator-prey interactions really accurately for the Simpson Desert, including for the introduced species there,” he said. “Foxes and cats, they’re introduced predators in Australia, and the algorithm could accurately pick what those species prey on.”
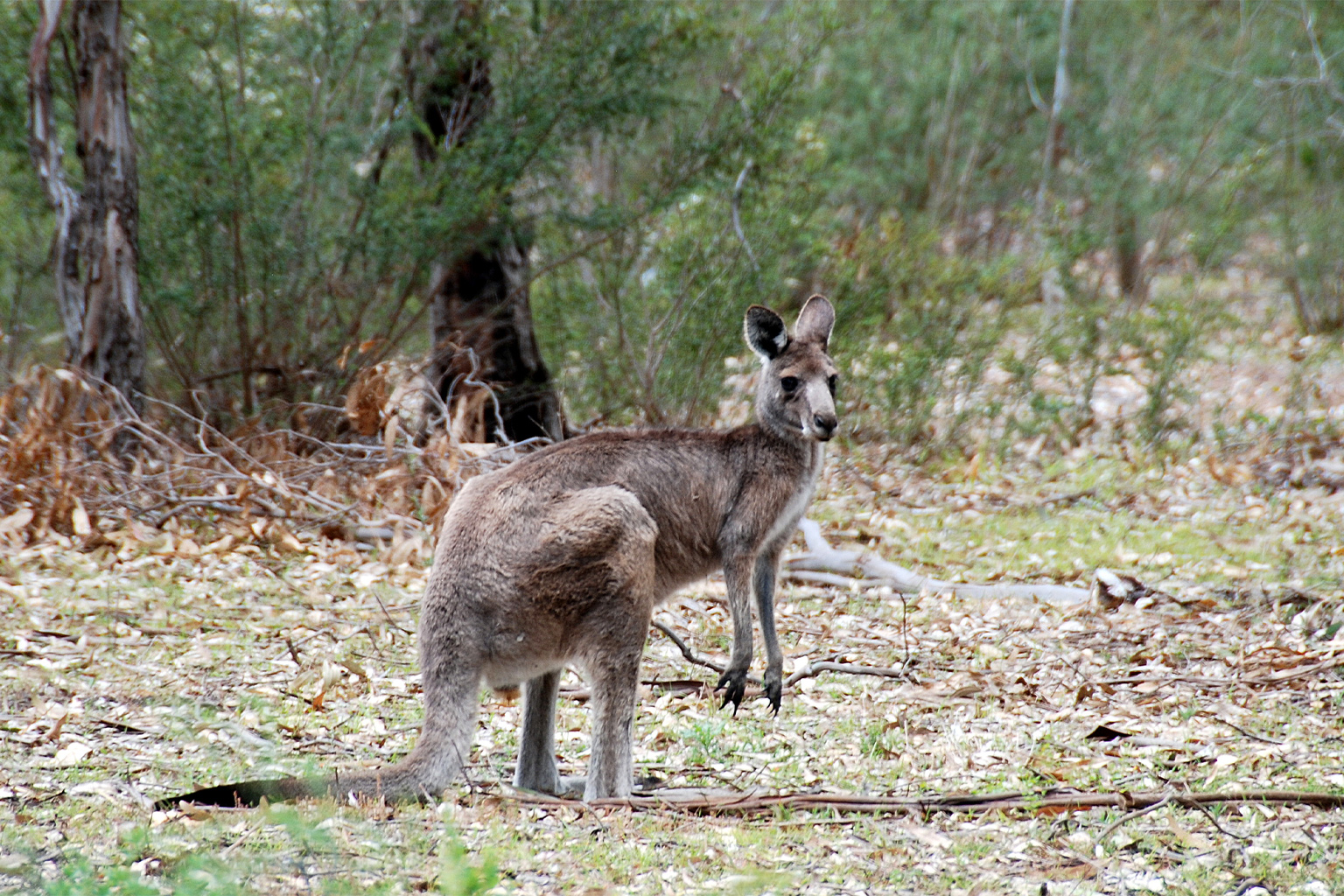
Llewelyn said the model, when used with other resources, could be a good tool for implementing conservation action on the ground.
He cited the example of red foxes (Vulpes vulpes), an invasive species introduced to Australia from Europe a century ago. The foxes are highly destructive to plants and native species in the country. However, until now, foxes have been prevented from establishing populations in the island state of Tasmania. Llewelyn said the model could be used to understand the ecological and biodiversity implications if the fox population was to proliferate in Tasmania.
“You could predict what species it would most likely prey on,” he said. “Then you can employ different conservation strategies targeted at those vulnerable native species. For example, you could train them to avoid the chemical cues of the foxes.”
However, given the paucity of data on species interactions, training the model is a tough task.
Llewelyn said the more data there are, the better the model’s predictions. “We know very little about species interactions, and what we know is only a tiny fraction of the interactions that are occurring out there,” he said.
While the training data are based on how species interact, the lack of data on which species are not interacting with each other could also skew the model’s predictions. “Just because it hasn’t been recorded doesn’t mean they don’t interact,” he said. “How to adjust your training data set so you are not including incorrect noninteractions, that is an area where this method could be improved.”
Llewelyn emphasized the need to incorporate other modeling approaches and combine all the results to get better predictions.
“You can use ensemble approaches where you bring together the predictions of various methods,” he said. “Now, it’s a bit fiddly because it’s all separate and you’ve got to run things separately, and then bring it together. But I am confident things will become more streamlined in the not-too-distant future, and then you can do ensemble modeling in one hit.”
Banner image: Red foxes (Vulpes vulpes) are highly destructive to plants and native species in the country. Image by xulescu_g via Flickr (CC BY-SA 2.0).
Abhishyant Kidangoor is a staff writer at Mongabay. Find him on Twitter @AbhishyantPK.
Machine learning helps researchers identify underground fungal networks
Citation:
Llewelyn, J., Strona, G., Dickman, C. R., Greenville, A. C., Wardle, G. M., Lee, M. S. Y., … Bradshaw, C. J. A. (2022). Predicting predator-prey interactions in terrestrial endotherms using random forest. Ecography. doi:10.1111/ecog.06619