- Scientists have used remote sensing, spectroscopy and machine learning to detect sick oak trees and distinguish between drought stress and oak wilt, a fungal disease.
- A recently published study describes how researchers established a link between physiological traits of trees and light reflectance to monitor the progression of symptoms in trees afflicted by oak wilt and drought.
- They used the data to build a predictive model that can identify symptoms and detect sick oaks 12 days before visual symptoms appear.
- Oaks are vital for climate regulation and carbon sequestration; however, the trees face threats to their survival because of a fatal fungal disease as well as the worsening impacts of climate change.
How do you identify sick oaks?
For a long time, detecting unhealthy oaks and identifying the disease afflicting them required a lot of manual labor. Scientists often looked out of airplanes or walked through forests in a bid to detect and find visible symptoms. Even then, one couldn’t really be sure.
New research attempts to find a solution to this long-standing problem.
A study published in the journal Proceedings of the National Academy of Sciences (PNAS) describes how a team of scientists used remote sensing, spectroscopy and machine learning to not only identify unhealthy oaks before visual symptoms appeared, but to also distinguish between drought stress and oak wilt, a deadly fungal disease.
The team monitored sick trees and, as symptoms progressed, observed physiological changes in them while also keeping an eye on how they reflected light. Once the researchers established a link between the two, they used the data to train a machine learning model that can now tell if an oak is sick, and if it suffers from drought stress or oak wilt.
“We obtained spectroscopic information in many wavelengths from light reflected from plants,” Jeannine Cavender-Bares, a co-author of the study and Distinguished McKnight University Professor of ecology, evolution and behavior at the University of Minnesota, told Mongabay in a video interview. “When we do this, we get a spectral fingerprint of the plant, which allows us to detect disease when we couple it with machine learning models.”
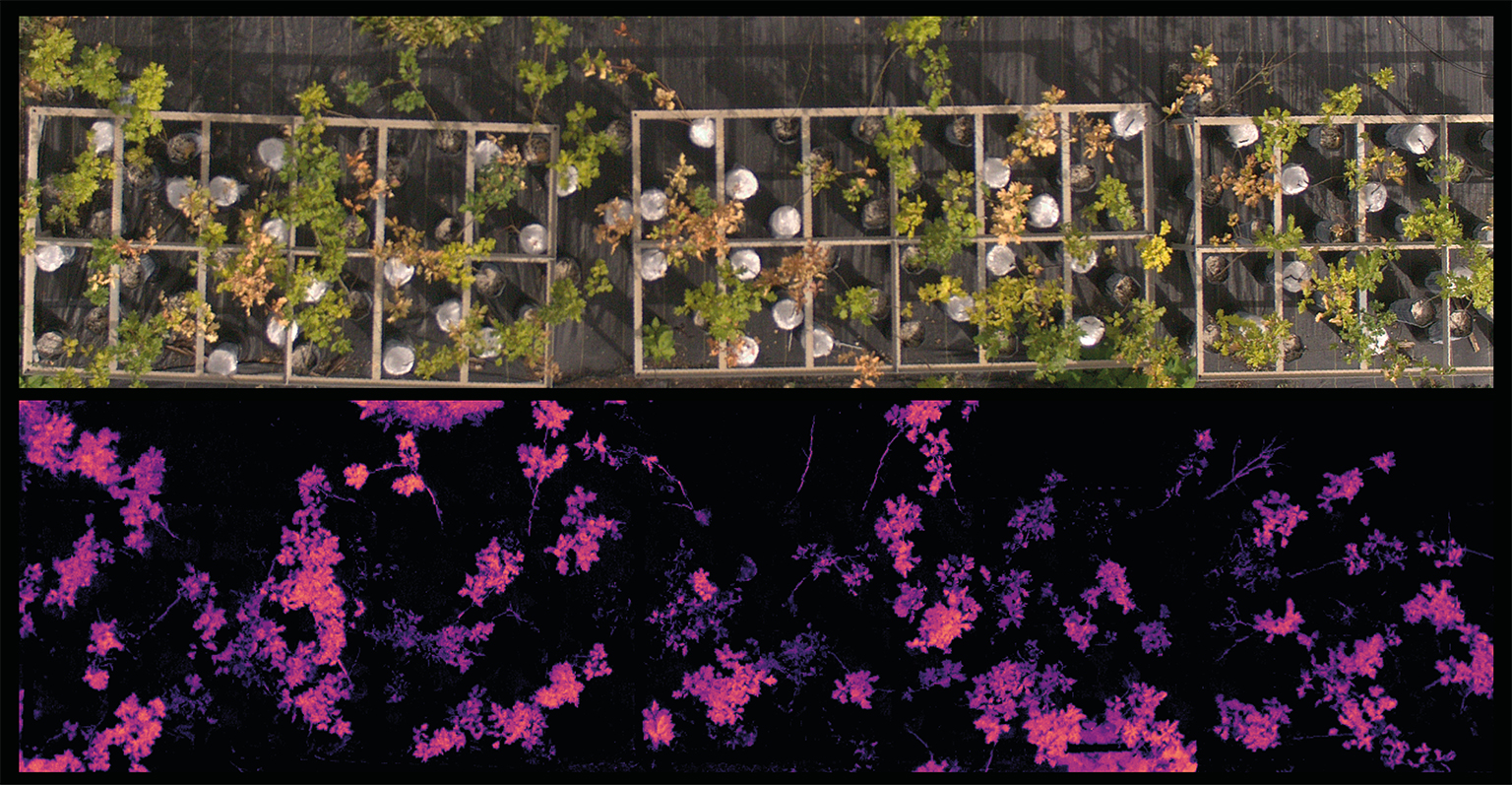
North America is home to the highest number of oak species in the world. According to the United States Forest Service, oaks comprise close to 11% of the total tree population in the country. Vital for climate regulation and carbon sequestration, oaks also support diverse biodiversity, filter pollutants from the air and prevent soil erosion.
However, these critical trees face an onslaught of threats. In particular, oak wilt, a fatal fungal disease, continues to ravage oak trees in the U.S. and Canada. Rising temperatures due to climate change also contribute to drought stress.
Early detection of oak wilt, and distinguishing it from drought stress symptoms, is key to protecting these trees. Once afflicted by oak wilt, it’s nearly impossible to save oaks. “The fungus goes down into the roots and into the vascular system,” Cavender-Bares said. “If undetected, it will kill all the neighbors, and then you’ve got a pocket of infested trees, which is harder to manage.”
Cavender-Bares and her team aim to address this gap with their work.
“We tried to get remote sensing signals tied to physiological processes that are happening within the plants,” Gerard Sapes, lead author of the study and research scientist at the University of Florida, told Mongabay in a video interview.
To get started, the team inoculated red oaks on the University of Minnesota campus with oak wilt fungus. A few trees were also subject to drought conditions. As the disease progressed, Sapes and his team measured a suite of physiological traits such as chlorophyll fluorescence, plant water content, and rehydration capacity while simultaneously gathering data on how the trees reflected light as the symptoms changed. This was done both at the leaf level by clamping a device on the leaf, as well by flying a sensor-attached drone over the top of the canopy.
“These two stressors have a similar effect on the tree. Basically, the leaves dry, and so there is a high chance of confusing a tree that is under drought with a tree that might be experiencing oak wilt,” Sapes said.
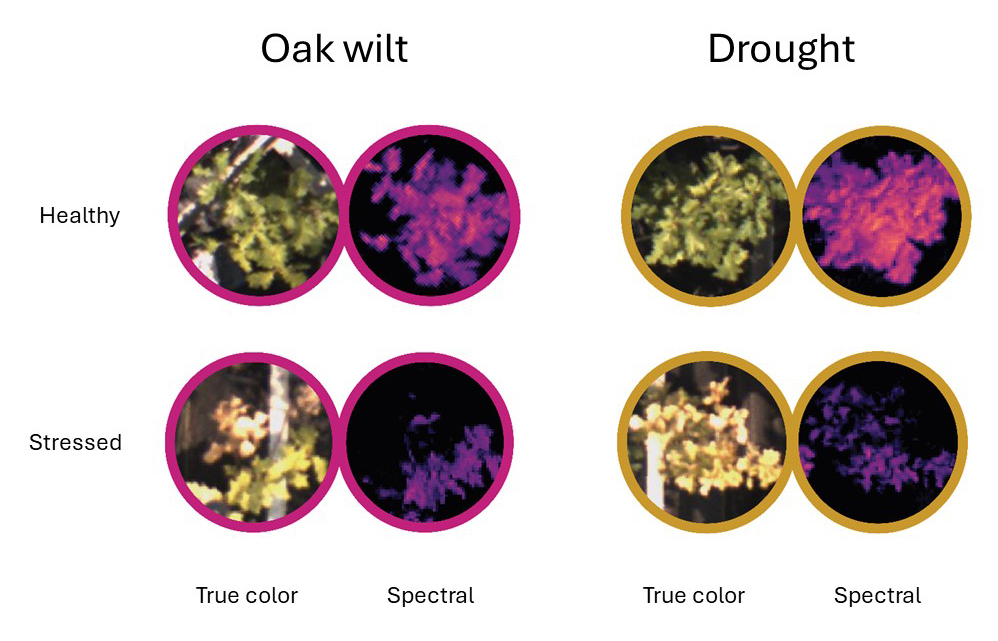
Both oak wilt and drought block water transportation to leaves through the tree’s xylem. However, oak wilt affected the conduits only in localized regions where the fungus was present. This was in contrast to trees subject to drought where the impaired pipes were not concentrated in any one region and were present all over.
“If the pipes that are nonfunctional are all lumped, chances are as you travel up to the tree, those connect to the same place in the canopy. The canopy’s patterns of stress mirror what’s happening inside, and in the case of oak wilt, the stress is concentrated in a few areas of the canopy,” Sapes said. “We can detect it by using a drone with a spectral signal that is sensitive to water and photosynthesis.”
The analysis of reflection patterns enabled the researchers to identify drops in the trees’ photosynthesis efficiency and rehydration capacity almost 12 days before visual symptoms appeared.
Using the data, the team then built a model that could take in remotely sensed spectral data and then predict physiological symptoms. When applied to a science reserve, the model was able to identify unhealthy oaks before any visual symptoms had appeared. “They looked like perfectly healthy plants,” Cavender-Bares said. “But the model was telling us, ‘No, this one is sick.’ And two weeks later, you could see it.”
The team now plans to further develop the model to include other tree diseases. They also aim to make it more widely available to ensure widespread use.
“We’re applying similar kinds of modeling approaches to other tree diseases by developing predictive maps,” Cavender-Bares said. “We want to make it available to people who need the information to do management work.”
Banner image: Acorns on an oak tree. Image by Mike Erskine via Unsplash (Public domain).
Abhishyant Kidangoor is a staff writer at Mongabay. Find him on 𝕏 @AbhishyantPK.
‘Immense potential’ in tech: Q&A with Wildlife Drones CEO Debbie Saunders
Citation:
Sapes, G., Schroeder, L., Scott, A., Clark, I., Juzwik, J., Montgomery, R. A., Guzmán Q, J. A., & Cavender-Bares, J. (2024). Mechanistic links between physiology and spectral reflectance enable previsual detection of oak wilt and drought stress. Proceedings of the National Academy of Sciences, 121(7). doi:10.1073/pnas.2316164121